Most asked Big Data Questions
Welcome to the Most asked Big Data Questions. Today we will cover some basic topics on SQL, Python, Machine learning and Case Study. Let’s get started with the Most asked Big Data Questions.
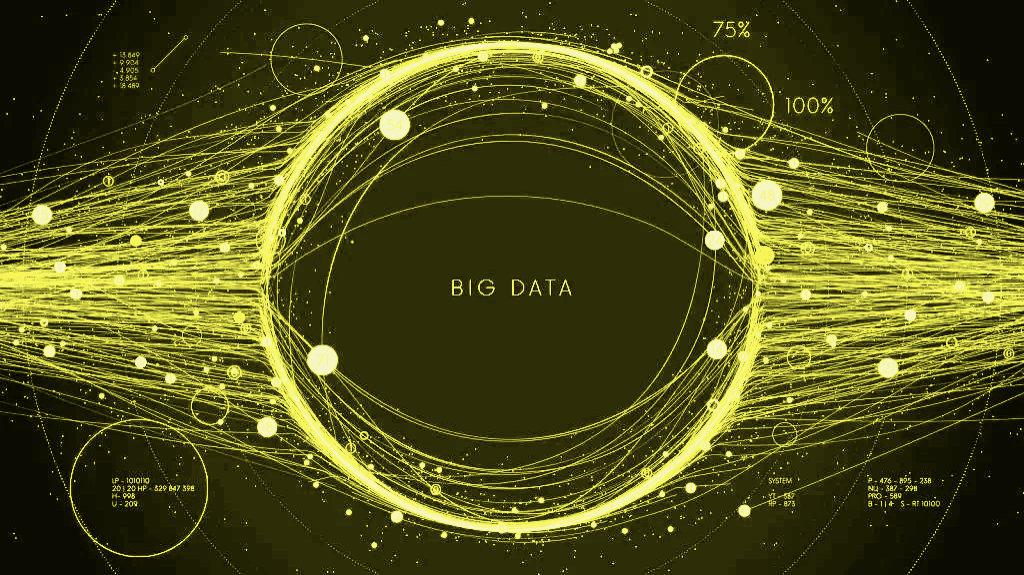
Videos to cover for Most asked Big Data Questions:
- In this section you will learn about Factorial and Armstrong in Python. Factorial and armstrong numbers are special numbers in mathematics and this will enhance your skills in python.
- For today’s sql lesson you should watch LAG() and LEAD() in SQL. In this video you will learn about LAG and LEAD functions in sql.
- Uniform and Logistic Distribution in Python| Part 8 | Complete Python Numpy Tutorial | The Data Monk. Watch this video to learn about uniform and logistic distribution in python.
- About today’s Machine learning work, watch Learn LSTM model in 30 minutes with complete code and theory that will teach you about the LSTM model with the complete code and theory.
- Next you are recommended to watch How Spotify Suggests Songs? Analytics/MBA Case Study | The Data Monk. This video will give you an insight of how your learnings are being used in the market for better outcomes.
To check your learnings head to:
- What is Scope Resolution in Python?
- What is the output of the below program?
- Define RDBMS and give example, also define the difference between Data Ware house and Database
- Explain the working of Map and Hash Map in Python
- How do you create a sample data of 1000 rows from a population of 1 million rows and 100 columns
The Data Monk services
We are well known for our interview books and have 70+ e-book across Amazon and The Data Monk e-shop page . Following are best-seller combo packs and services that we are providing as of now
- YouTube channel covering all the interview-related important topics in SQL, Python, MS Excel, Machine Learning Algorithm, Statistics, and Direct Interview Questions
Link – The Data Monk Youtube Channel - Website – ~2000 completed solved Interview questions in SQL, Python, ML, and Case Study
Link – The Data Monk website - E-book shop – We have 70+ e-books available on our website and 3 bundles covering 2000+ solved interview questions. Do check it out
Link – The Data E-shop Page - Instagram Page – It covers only Most asked Questions and concepts (100+ posts). We have 100+ most asked interview topics explained in simple terms
Link – The Data Monk Instagram page - Mock Interviews/Career Guidance/Mentorship/Resume Making
Book a slot on Top Mate
The Data Monk e-books
We know that each domain requires a different type of preparation, so we have divided our books in the same way:
1. 2200 Interview Questions to become Full Stack Analytics Professional – 2200 Most Asked Interview Questions
2.Data Scientist and Machine Learning Engineer -> 23 e-books covering all the ML Algorithms Interview Questions
3. 30 Days Analytics Course – Most Asked Interview Questions from 30 crucial topics
You can check out all the other e-books on our e-shop page – Do not miss it
For any information related to courses or e-books, please send an email to [email protected]