Why do we predict?
We predict in order to identify the trend of the future by using our sample data set. Whenever we create a model, we try to create a formula out of our sample data set. And the aim of this formula is to satisfy all the possible conditions of the universe.
Mathematicians and Statisticians all across the globe try to create a perfect model that can answer future questions.
Thus we create a model, and this model is bound to have some error. Why? Because we can’t cover all the possible combinations to fit in one formula. The error or difference between the actual and predicted value is called prediction error.
Bias – It is the difference between the average prediction of the model with the actual values. A model with HIGH bias will create a very simple model and it will be far away from the actual values in both train and test data set
Examples of low-bias machine learning algorithms include: Decision Trees, k-Nearest Neighbors and SVM.
Examples of high-bias machine learning algorithms include: Linear Regression, Linear Discriminant Analysis and Logistic Regression
Variance – Variance refers to the spread of our data. A model with high variance will be so specific in its training dataset that it tries to cover all the points while training the data which results in high training accuracy but low test accuracy
Examples of low-variance machine learning algorithms include: Linear Regression, Linear Discriminant Analysis and Logistic Regression.
Examples of high-variance machine learning algorithms include: Decision Trees, k-Nearest Neighbors and Support Vector Machines.
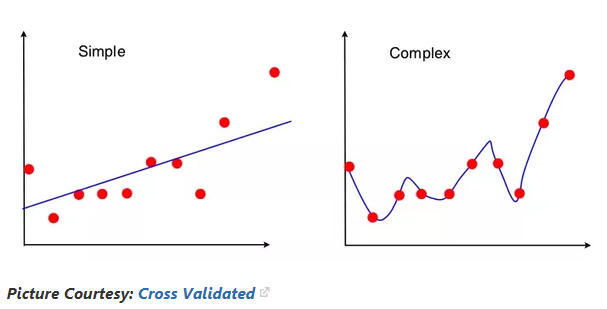
As you can see, the line in the left tries to cover all the points, so it creates a complicated model which is very accurate in the training data set.
Let’s see how an under fitting, over fitting, and good model looks like
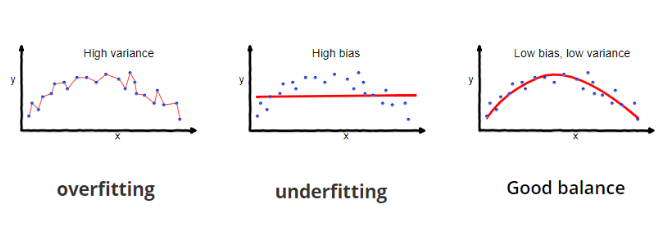
As you can see, A high variance occurs in a model that tries to create a complicated formula on the training data set.
A high bias model is very generic. Matlab aiwaiey kuch v average bna diya
If you want to understand the mathematics behind these errors, then below is the formula
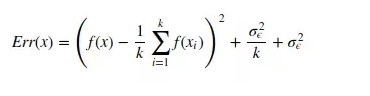
The above formula has 3 terms, the first term is the bias square, second is the variance and third is the irreducible error.
No matter what, you can’t remove the irreducible error. It is the measure of noise in the data and you can’t have a noiseless data set.
When you have a very limited dataset then there is a high chance of getting a under-fitting data set(High Bias and Low Variance)
When you have very noisy data then the model tries to fit in a complicated model which might result in over-fitting on the training dataset(High Variance and Low Bias)
What is the bias-variance trade-off?
The trade-off between bias and variance is done to minimize the overall error(formula above)
Error = Reducible Error+Irreducible Error
Reducible error = (Bias)^2 + Variance

Let’s try to ease out the formula for Bias and Variance
Bias =Estimation of target-target
Variance of estimates = (Target – Estimated target)^2
The variance error measure how much our target function would differ if a new training data was used.
To keep all the errors positive, we have bias square, variance(which itself is a squared value) and irreducible error squared
The bias–variance tradeoff is the property of a set of predictive models whereby models with a lower bias in parameter estimation have a higher variance of the parameter estimates across samples, and vice versa.
How do we actually try to make bias-variance trade-off?
There are multiple methods for B-V Trade-off
-Separate training and testing dataset
-Cross-Validation
-Good Performance metrics
-Fitting model parameters
Keep Learning 🙂
The Data Monk
The Data Monk services
We are well known for our interview books and have 70+ e-book across Amazon and The Data Monk e-shop page . Following are best-seller combo packs and services that we are providing as of now
- YouTube channel covering all the interview-related important topics in SQL, Python, MS Excel, Machine Learning Algorithm, Statistics, and Direct Interview Questions
Link – The Data Monk Youtube Channel - Website – ~2000 completed solved Interview questions in SQL, Python, ML, and Case Study
Link – The Data Monk website - E-book shop – We have 70+ e-books available on our website and 3 bundles covering 2000+ solved interview questions. Do check it out
Link – The Data E-shop Page - Instagram Page – It covers only Most asked Questions and concepts (100+ posts). We have 100+ most asked interview topics explained in simple terms
Link – The Data Monk Instagram page - Mock Interviews/Career Guidance/Mentorship/Resume Making
Book a slot on Top Mate
The Data Monk e-books
We know that each domain requires a different type of preparation, so we have divided our books in the same way:
1. 2200 Interview Questions to become Full Stack Analytics Professional – 2200 Most Asked Interview Questions
2.Data Scientist and Machine Learning Engineer -> 23 e-books covering all the ML Algorithms Interview Questions
3. 30 Days Analytics Course – Most Asked Interview Questions from 30 crucial topics
You can check out all the other e-books on our e-shop page – Do not miss it
For any information related to courses or e-books, please send an email to [email protected]