Multicollinearity in Simple Terms
We all know the definition of multi-collinearity i.e. when 2 or more explanatory variable in multi regression model are highly linearly related then it’s called multicollinearity
Example –
Age and Selling price of a Car
Education and Annual Income
Height and Weight
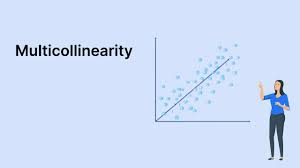
Why should we remove multicollinearity from our model?
Example, You are watching WWE and Batista is thrashing Undertaker. Now you know that Batista is better.
But suppose it’s a Royal Rumble where 5 wrestlers are beating Undertaker simultaneously. Now, you can’t say which one is attacking with what intensity and thus you can’t say which wrestler among the five are better.
Thus when you have multiple variables which are correlated, the model is unable to give proper weightage about the impact of each variable. So, we need to remove redundant variables
What all methods are used to remove multi-collinearity?
There are two methods to do the same:-
1. VIF – It stands for Variance Inflation Factor. During regression analysis, VIF assesses whether factors are correlated to each other (multicollinearity), which could affect p-values and the model isn’t going to be as reliable
Factor with high VIF should be removed. A VIF of 1 suggests no correlation
2. PCA – Principal component analysis (PCA) is a technique used to emphasise variation and bring out strong patterns in a dataset. It’s often used to make data easy to explore and visualise.
How so we deal with Multicollinearity in our model?
1. You can use feature engineering to convert the two variables into one and then use this variable
2. Use VIF/PCA to eliminate one of the variables
You should eliminate the one which is not strongly correlated with the target variable
I think this is much about Multi-collinearity. Let me know if you have any questions
Keep Learning 🙂
The Data Monk
The Data Monk services
We are well known for our interview books and have 70+ e-book across Amazon and The Data Monk e-shop page . Following are best-seller combo packs and services that we are providing as of now
- YouTube channel covering all the interview-related important topics in SQL, Python, MS Excel, Machine Learning Algorithm, Statistics, and Direct Interview Questions
Link – The Data Monk Youtube Channel - Website – ~2000 completed solved Interview questions in SQL, Python, ML, and Case Study
Link – The Data Monk website - E-book shop – We have 70+ e-books available on our website and 3 bundles covering 2000+ solved interview questions. Do check it out
Link – The Data E-shop Page - Instagram Page – It covers only Most asked Questions and concepts (100+ posts). We have 100+ most asked interview topics explained in simple terms
Link – The Data Monk Instagram page - Mock Interviews/Career Guidance/Mentorship/Resume Making
Book a slot on Top Mate
The Data Monk e-books
We know that each domain requires a different type of preparation, so we have divided our books in the same way:
1. 2200 Interview Questions to become Full Stack Analytics Professional – 2200 Most Asked Interview Questions
2.Data Scientist and Machine Learning Engineer -> 23 e-books covering all the ML Algorithms Interview Questions
3. 30 Days Analytics Course – Most Asked Interview Questions from 30 crucial topics
You can check out all the other e-books on our e-shop page – Do not miss it
For any information related to courses or e-books, please send an email to [email protected]