Suppose you have 1000 rows of data and you want to make a model to predict whether a person is suffering from Thyroid. There are 15 columns with 15th one being a binary variable of 0(Normal) and 1 (Thyroid).
Firstly you have to figure out the model which you want to make and then you need to get the important features for the model. Once you have these, you will have to train your model. This is the time when you have to decide the number of rows you want to use for training the dataset.
Typically, the training dataset is 70-80% of the total. When you provide these data to your model, the model will start making rules accordingly.
For ex. Suppose we have weight, blood sugar, and waist size as the important features. If there are people with Thyroid and attributes like [100, 300, 42] where 100 is the weight in kilograms, 300 is the blood sugar level and 42 is the waist size. If this row is selected in the training dataset then the model will create a rule saying that high weight, blood sugar level, and large waist size results in Thyroid. We will have some 700-800 such rows in our training dataset to make multiple rules.
The dataset on which you train your model or the dataset which the model uses to make rules are called training dataset.
We started with 1000 rows and trained our model on 750 rows. Now the rest 250 rows will be used to test the accuracy of the model. See, we already have the output of these 250 rows, but we have to feed the data to our model without the output column and this way it will start applying the rules created so far from the training dataset. Now you will have 16 columns in your test dataset where the 16th one is the predicted values. The 15th and 16th column contains the actual and predicted values. So, you can create a confusion matrix to understand the accuracy and performance of your model.
Bottom line is that you divide the total dataset into 80:20 or 70:30 or anything around this (train:test) and then build your model on the larger chunk of data and check it against the smaller one. Once you have the result for testing the data, then make a confusion matrix to understand the result.
The code in Python to split the complete dataset into train and test is given below
from sklearn.model_selection import train_test_split
X_train, X_test, y_train, y_test = train_test_split(X, y, test_size = 0.25)
train_test_split is a function present in the sklearn.model_selection package. In the train_test_split() function you need to provide 3 parameters.
X denotes a dataset with all the rows and independent variables, y is the outcome of the dependent variable(Thyroid in this case) and test_size is 0.25 i.e. Training dataset will have 75% rows and the test size will be 25%.
This function will create 4 datasets i.e. X_train, y_train, X_test, and y_test.
X_train – 75% of the data and 14 columns (excluded Outcome column)
y_train – 25% of the data with only one column i.e. Output column
X_test – 75% of the data with the same columns as X_train
y_test – Contains the result with which you have to match the model’s result
Now let’s talk about the confusion matrix in brief
A confusion matrix is a summary of prediction results on a classification problem.
The number of correct and incorrect predictions are summarized with count values and broken down by each class. This is the key to the confusion matrix.
The confusion matrix shows the ways in which your classification model
is confused when it makes predictions.
It gives you insight not only into the errors being made by your classifier but more importantly the types of errors that are being made.
It is this breakdown that overcomes the limitation of using classification accuracy alone.
In a two-class problem, we are often looking to discriminate between observations with a specific outcome, from normal observations.
Such as a disease state or event from no disease state or no event.
In this way, we can assign the event row as “positive” and the no-event row as “negative“. We can then assign the event column of predictions as “true” and the no-event as “false“.
This gives us:
- “true positive” for correctly predicted event values.
- “false positive” for incorrectly predicted event values.
- “true negative” for correctly predicted no-event values.
- “false negative” for incorrectly predicted no-event values.
A confusion matrix is typically computed in any machine learning classifier such us logistic regression, decision tree, support vector machine, naive bayes etc. to calculate a cross-tabulation of observed (true) and predicted classes (model). There are several metrics such as precision and recall that helps us interpret the accuracy of the model and choose the best model.
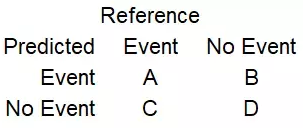
Sensitivity = A/(A+C)
Specificity = D/(B+D)
Prevalence = (A+C)/(A+B+C+D)
Positive Predicted Value (PPV) =
(sensitivity * prevalence)/((sensitivity*prevalence) + ((1-specificity)*(1-prevalence)))
Negative Predicted Value (NPV) =
(specificity * (1-prevalence))/(((1-sensitivity)*prevalence) + ((specificity)*(1-prevalence)))
Detection Rate = A/(A+B+C+D)
Detection Prevalence = (A+B)/(A+B+C+D)
Balanced Accuracy = (sensitivity+specificity)/2
Precision = A/(A+B)
Recall = A/(A+C)
We are using the Thyroid example to understand how this confusion matrix is important to us. Suppose our test data set has 100 rows and the values in the Confusion matrix are
true positive – 45
false positive – 5
true negative– 5
false negative – 45
So, the accuracy of your model will be (45+45)/(45+5+5+45) i.e. number of
correct prediction divided by total prediction which is 90%.
False positive shows that there were 5 people who did not have Thyroid but our model projected it as suffering from it.
To learn a lot more about what interview questions are asked in Data Science interviews(Myntra, Flipkart, Accenture, Bookmyshow, Oyo, etc.), you can go through our best seller
What do they ask in Data Science interview
What do they ask in Data Science interview Part 2
Keep coding 🙂
XtraMous
The Data Monk services
We are well known for our interview books and have 70+ e-book across Amazon and The Data Monk e-shop page . Following are best-seller combo packs and services that we are providing as of now
- YouTube channel covering all the interview-related important topics in SQL, Python, MS Excel, Machine Learning Algorithm, Statistics, and Direct Interview Questions
Link – The Data Monk Youtube Channel - Website – ~2000 completed solved Interview questions in SQL, Python, ML, and Case Study
Link – The Data Monk website - E-book shop – We have 70+ e-books available on our website and 3 bundles covering 2000+ solved interview questions. Do check it out
Link – The Data E-shop Page - Instagram Page – It covers only Most asked Questions and concepts (100+ posts). We have 100+ most asked interview topics explained in simple terms
Link – The Data Monk Instagram page - Mock Interviews/Career Guidance/Mentorship/Resume Making
Book a slot on Top Mate
The Data Monk e-books
We know that each domain requires a different type of preparation, so we have divided our books in the same way:
1. 2200 Interview Questions to become Full Stack Analytics Professional – 2200 Most Asked Interview Questions
2.Data Scientist and Machine Learning Engineer -> 23 e-books covering all the ML Algorithms Interview Questions
3. 30 Days Analytics Course – Most Asked Interview Questions from 30 crucial topics
You can check out all the other e-books on our e-shop page – Do not miss it
For any information related to courses or e-books, please send an email to [email protected]