We know that each domain requires a different type of preparation, so we have divided our books in the same way:
Our best seller:
✅ Become a Full Stack Analytics Professional with The Data Monk’s master e-book with 2200+ interview questions covering 23 topics – 2200 Most Asked Interview Questions
Machine Learning e-book
✅ Data Scientist and Machine Learning Engineer -> 23 e-books covering all the ML Algorithms Interview Questions
Domain wise interview e-books
✅ Data Analyst and Product Analyst Interview Preparation -> 1100+ Most Asked Interview Questions
✅ Business Analyst Interview Preparation -> 1250+ Most Asked Interview Questions
The Data Monk – 30 Days Mentorship program
We are a group of 30+ people with ~8 years of Analytics experience in product-based companies. We take interviews on a daily basis for our organization and we very well know what is asked in the interviews.
Other skill enhancer websites charge 2lakh+ GST for courses ranging from 10 to 15 months.
We only focus on making you a clear interview with ease. We have released our Become a Full Stack Analytics Professional for anyone in 2nd year of graduation to 8-10 YOE. This book contains 23 topics and each topic is divided into 50/100/200/250 questions and answers. Pick the book and read it thrice, learn it, and appear in the interview.
We also have a complete Analytics interview package
– 2200 questions ebook (Rs.1999) + 23 ebook bundle for Data Science and Analyst role (Rs.1999)
– 4 one-hour mock interviews, every Saturday (top mate – Rs.1000 per interview)
– 4 career guidance sessions, 30 mins each on every Sunday (top mate – Rs.500 per session)
– Resume review and improvement (Top mate – Rs.500 per review)
Snapdeal Data Science Interview Questions
Snapdeal, is an Indian e-commerce company based in New Delhi, India. The company was founded by Kunal Bahl and Rohit Bansal in February 2010. It is one of the most trusted online shopping platforms around the globe.
Snapdeal Data Science Interview Questions
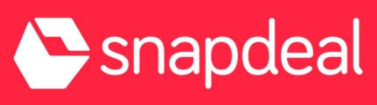
Interview Process
The Snapdeal Data Science interview process typically consists of 5 rounds, each designed to evaluate different aspects of your technical and analytical skills:
Round 1 – Technical round
Focus: Basic understanding of Data Science concepts, SQL, and Python/R.
Format: You’ll be asked to explain your projects and solve a few coding or SQL problems.
Round 2 – Walk-in/Face-to-Face Technical Round
Focus: Advanced SQL, coding, and problem-solving.
Format: You’ll solve problems on a whiteboard or shared document.
Round 3 – Project Analysis
Focus: Deep dive into your past projects.
Format: You’ll be asked to explain your approach, tools used, and the impact of your work.
Round 4 – Case Studies
Focus: Business problem-solving and data-driven decision-making.
Format: You’ll be given a real-world scenario and asked to propose solutions.
Round 5 – Hiring Manager Round
Focus: Cultural fit, communication skills, and long-term career goals.
Format: Behavioral questions and high-level discussions about your experience.
Difficulty of Questions
Here’s a breakdown of the difficulty level for each topic:
SQL: 8/10 – Practice advanced queries, joins, and optimization techniques.
Python/R: 9/10 – Focus on data manipulation, libraries, and machine learning implementations.
Statistics/ML: High – Be thorough with probability, hypothesis testing, and model evaluation.
Case Studies: Moderate – Practice solving business problems with a structured approach.
Snapdeal Interview Questions
1. If you are having 3GB RAM in your machine and you want to train your model on an 8GB dataset. How would you go about this problem?
Answer:
To handle this situation, you can use the following techniques:
Batch Processing: Split the dataset into smaller batches and train the model incrementally.
Out-of-Core Learning: Use libraries like Dask or Vaex that process data in chunks without loading the entire dataset into memory.
Data Sampling: Train the model on a representative sample of the dataset.
Cloud Computing: Use cloud platforms (e.g., AWS, Google Cloud) with higher memory capacity.
Feature Reduction: Remove irrelevant features to reduce dataset size.
2. How can you tell if a given coin is biased?
Answer:
We can use hypothesis testing to determine if a coin is biased:
Null Hypothesis (H₀): The coin is fair (probability of heads p=0.5).
Alternative Hypothesis (H₁): The coin is biased ( p ≠ 0.5 ).
Experiment: Flip the coin n times and record the number of heads.
Test Statistic: Use the binomial test or chi-square test to calculate the p-value.
Conclusion: If the p-value < significance level (e.g., 0.05), reject H₀ and conclude the coin is biased.
3. Why does L1 regularization cause parameter sparsity whereas L2 regularization does not?
Answer:
L1 Regularization: Adds the absolute value of coefficients to the loss function. It tends to shrink less important features to exactly zero, resulting in sparse models.
L2 Regularization: Adds the squared value of coefficients to the loss function. It shrinks coefficients but rarely reduces them to zero, preserving all features.
Example:
L1: Useful for feature selection (e.g., identifying key customer attributes).
L2: Useful when all features are relevant (e.g., predicting house prices).
4. Explain a probability distribution that is not normal and how to apply that.
Answer:
Poisson Distribution: Models the number of events occurring in a fixed interval (e.g., customer arrivals per hour).
Application:
Use case: Predicting the number of orders Snapdeal receives in an hour.
The Poisson distribution formula is: P(X = x) = (e^-λ * λ^x) / x!.
Where:
P(X = x): Probability of getting exactly ‘x’ occurrences.
λ: (lambda): Average rate of occurrences.
e: Euler’s number (approximately 2.718).
x!: Factorial of x.
Example: If Snapdeal averages 50 orders/hour, the probability of receiving 60 orders is P(X=60).
5. There are 6 marbles in a bag, 1 is white. You reach in the bag 100 times. After drawing a marble, it is placed back in the bag. What is the probability of drawing the white marble at least once?
Answer:
Probability of not drawing the white marble in one attempt: 5/6
Probability of not drawing it in 100 attempts: (5/6)^100
Probability of drawing it at least once: 1 – (5/6)^100 ≈ 1 – 0.000021 = 0.999979
Conclusion: There’s a 99.9979% chance of drawing the white marble at least once.
6. What is the importance of Markov Chains in Data Science?
Answer:
Definition: A stochastic model describing a sequence of events where the probability of each event depends only on the previous state.
Applications:
Customer Journey Analysis: Predict transitions between states (e.g., browsing → cart → purchase).
Recommendation Systems: Model user behavior patterns.
Fraud Detection: Identify unusual sequences of transactions.
7. If the model isn’t perfect, how would you select the threshold so that the model outputs 1 or 0 for a label?
Answer:
ROC Curve: Plot True Positive Rate (TPR) vs. False Positive Rate (FPR) for different thresholds.
Optimal Threshold: Choose the threshold that maximizes TPR while minimizing FPR.
Business Context: Adjust the threshold based on the cost of false positives/negatives.
Example: In fraud detection, a lower threshold may be preferred to catch more frauds, even if it increases false positives.
8. Can you explain the concept of a false positive and false negative? Give some examples to make things clear.
Answer:
False Positive (Type I Error): Incorrectly predicting a positive outcome (e.g., flagging a legitimate transaction as fraud).
False Negative (Type II Error): Incorrectly predicting a negative outcome (e.g., failing to flag a fraudulent transaction).
Example:
Medical Testing:
False Positive: Healthy person diagnosed with a disease.
False Negative: Sick person diagnosed as healthy.
9. Prior to building any kind of model, why do we need to complete the feature selection step? What will happen if we skip it?
Answer:
Purpose of Feature Selection:
- Reduces overfitting by removing irrelevant features.
- Improves model interpretability and performance.
- Reduces training time and computational cost.
Consequences of Skipping:
- Increased risk of overfitting.
- Poor model performance due to noise from irrelevant features.
- Longer training times and higher resource usage.
10. Suggest some ways through which you can detect anomalies in a given dataset.
Answer:
Statistical Methods:
- Z-score: Flag data points with |Z| > 3.
- IQR: Identify outliers outside Q1 – 1.5 × IQR or Q3 + 1.5 × IQR.
Machine Learning:
- Isolation Forest: Detects anomalies by isolating data points.
- One-Class SVM: Identifies outliers in unsupervised data.
Visualization:
- Box plots, scatter plots, or heatmaps to spot anomalies.
Rule-Based:
- Flag transactions above a threshold (e.g., ₹50,000).
The Data Monk services
We are well known for our interview books and have 70+ e-book across Amazon and The Data Monk e-shop page . Following are best-seller combo packs and services that we are providing as of now
- YouTube channel covering all the interview-related important topics in SQL, Python, MS Excel, Machine Learning Algorithm, Statistics, and Direct Interview Questions
Link – The Data Monk Youtube Channel - Website – ~2000 completed solved Interview questions in SQL, Python, ML, and Case Study
Link – The Data Monk website - E-book shop – We have 70+ e-books available on our website and 3 bundles covering 2000+ solved interview questions. Do check it out
Link – The Data E-shop Page - Instagram Page – It covers only Most asked Questions and concepts (100+ posts). We have 100+ most asked interview topics explained in simple terms
Link – The Data Monk Instagram page - Mock Interviews/Career Guidance/Mentorship/Resume Making
Book a slot on Top Mate
For any information related to courses or e-books, please send an email to [email protected]