We know that each domain requires a different type of preparation, so we have divided our books in the same way:
Our best seller:
✅Become a Full Stack Analytics Professional with The Data Monk’s master e-book with 2200+ interview questions covering 23 topics – 2200 Most Asked Interview Questions
Machine Learning e-book
✅Data Scientist and Machine Learning Engineer ->23 e-books covering all the ML Algorithms Interview Questions
Domain wise interview e-books
✅Data Analyst and Product Analyst Interview Preparation ->1100+ Most Asked Interview Questions
✅Business Analyst Interview Preparation ->1250+ Most Asked Interview Questions
The Data Monk – 30 Days Mentorship program
We are a group of 30+ people with ~8 years of Analytics experience in product-based companies. We take interviews on a daily basis for our organization and we very well know what is asked in the interviews.
Other skill enhancer websites charge 2lakh+ GST for courses ranging from 10 to 15 months.
We only focus on making you a clear interview with ease. We have released our Become a Full Stack Analytics Professional for anyone in 2nd year of graduation to 8-10 YOE. This book contains 23 topics and each topic is divided into 50/100/200/250 questions and answers. Pick the book and read it thrice, learn it, and appear in the interview.
We also have a complete Analytics interview package
– 2200 questions ebook (Rs.1999) + 23 ebook bundle for Data Science and Analyst role (Rs.1999)
– 4 one-hour mock interviews, every Saturday (top mate – Rs.1000 per interview)
– 4 career guidance sessions, 30 mins each on every Sunday (top mate – Rs.500 per session)
– Resume review and improvement (Top mate – Rs.500 per review)
YouTube channel covering all the interview-related important topics in SQL, Python, MS Excel, Machine Learning Algorithm, Statistics, and Direct Interview Questions
Link –The Data Monk Youtube Channel
E-bay Most Asked Data Science Interview Questions
Company: eBay
Designation: Data Scientist
Year of Experience Required: 0 to 4 years
Technical Expertise: SQL, Python/R, Statistics, Machine Learning, Case Studies
Salary Range: 15LPA – 30LPA
eBay Inc., founded in 1995 by Pierre Omidyar, is a global e-commerce leader headquartered in San Jose, California. Known for its consumer-to-consumer and business-to-consumer sales platform, eBay is a pioneer in online marketplaces. If you’re preparing for a Data Science role at eBay, here’s a detailed breakdown of their interview process and the types of questions you can expect.
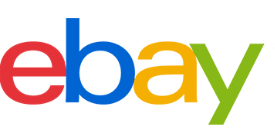
Interview Process
The eBay Data Science interview process typically consists of 5 rounds, each designed to evaluate different aspects of your technical and analytical skills:
Round 1 – Telephonic Screening
Focus: Basic understanding of Data Science concepts, SQL, and Python/R.
Format: You’ll be asked to explain your projects and solve a few coding or SQL problems.
Round 2 – Walk-in/Face-to-Face Technical Round
Focus: Advanced SQL, coding, and problem-solving.
Format: You’ll solve problems on a whiteboard or shared document.
Round 3 – Project Analysis
Focus: Deep dive into your past projects.
Format: You’ll be asked to explain your approach, tools used, and the impact of your work.
Round 4 – Case Studies
Focus: Business problem-solving and data-driven decision-making.
Format: You’ll be given a real-world scenario and asked to propose solutions.
Round 5 – Hiring Manager Round
Focus: Cultural fit, communication skills, and long-term career goals.
Format: Behavioral questions and high-level discussions about your experience.
Difficulty of Questions
SQL – 9/10
1) Find all the students who either are male or live in Mumbai have Mumbai as a part of their address.
Select name
From students
Where lower(gender)in(‘male’,’m’)
Or lower(address)=‘%mumbai%'
2) Suppose there are two columns in employee table i.e. empid and email. Get all the unique domains like gmail.com, yahoo.com, outlook.com, etc.
select substr(email, instr(email, ‘@’) + 1, length(email)) as Domain
from emp1;
3) Can you join two tables without any common columns?
Yes, we can use a CROSS JOIN without any common columns. For example, if we have ‘RollNumber’ and ‘NameofStudents’ in TableA, and their ‘Class’ (let’s say 5th) in TableB
We will use cross join to append class against each student.
SELECT B.CLASS, A.ID, A.NAME
FROM A, B
WHERE 1=1
4) Given an ’employees’ table with columns ’employee_id’, ’employee_name’, ‘department_id’, and ‘salary’, write a MySQL query to find the employee with the highest salary in each department. Display the department_id, employee_name, and salary.
SELECT e.department_id, e.employee_name, e.salary FROM employees e WHERE e.salary = ( SELECT MAX(salary) FROM employees WHERE department_id = e.department_id );
5) Given a ‘users’ table with columns ‘user_id’, ‘username’, and ’email’, write a MySQL query to find all email addresses that appear more than once in the table. Display the duplicate email addresses
SELECT email FROM users GROUP BY email HAVING COUNT(email) > 1;
R/Python – 7/10
1) Write a Python function that takes an integer as input and returns the sum of its digits.
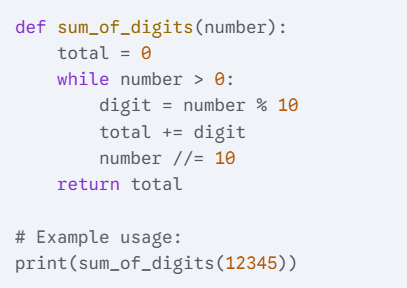
2) Write a Python function that takes a list of numbers as input and returns True
if the list is sorted in ascending order, and False
otherwise.
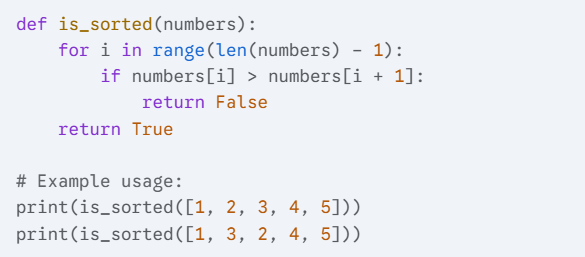
3) Write a Python function that takes a string as input and returns the first non-repeating character in the string. If there are no non-repeating characters, return None
.
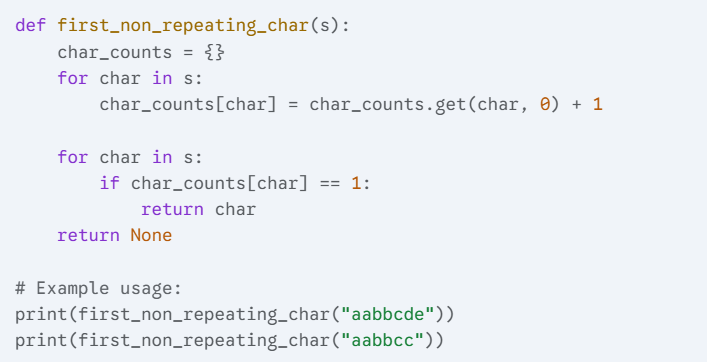
4) Write a Python function that generates the Fibonacci sequence up to a given number of terms.
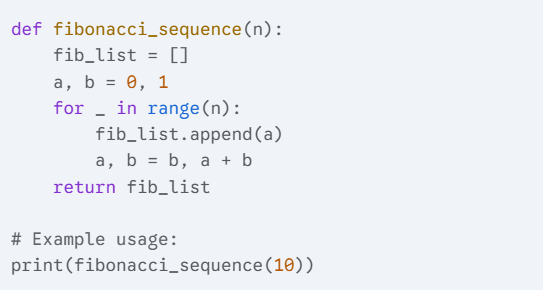
5) Write a simple text-based adventure game logic. For example, a function that takes a user’s choice (e.g., “left”, “right”) and returns a string describing the outcome.
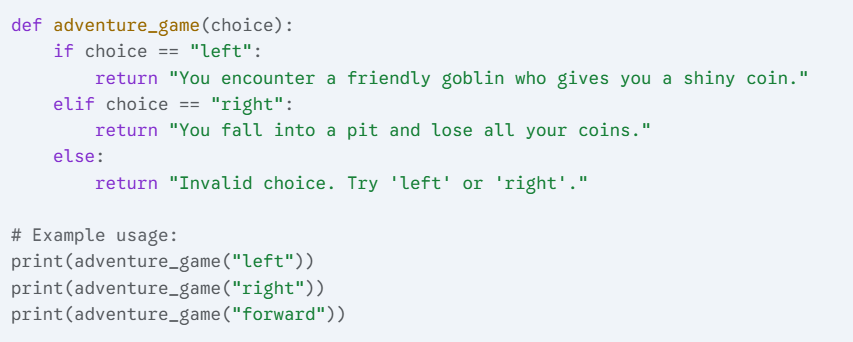
Statistics/ML
1) What are the assumptions required for linear regression? What if some of these assumptions are violated?
Linear regression assumes:
- Linearity – Relationship between independent and dependent variables is linear.
- Independence – Observations are independent of each other.
- Homoscedasticity – Constant variance of residuals across all levels of independent variables.
- Normality of Errors – Residuals should be normally distributed.
- No Multicollinearity – Independent variables should not be highly correlated.
Impact of Violations:
Multicollinearity: Use PCA or remove correlated features.
Non-linearity: Model will be inaccurate; transformation or polynomial regression may help.
Non-independence: Time-series modeling or clustering might be needed.
Heteroscedasticity: Use weighted regression or transform data.
Non-normality of errors: Large datasets reduce impact; otherwise, transformations help.
2) Why are long-tailed distributions important in classification and regression problems?
Long-tailed distributions occur when a few categories dominate the data while many others appear infrequently.
Importance:
- In classification, rare categories may be underrepresented, causing bias.
- In regression, extreme values can heavily influence predictions.
Examples:
- E-commerce: Few popular products sell a lot, while many niche products have low sales.
- Fraud detection: Fraudulent transactions are rare but crucial to detect.
- Language models: Common words dominate, but rare words matter for meaning.
Handling methods:
- Use log transformations to reduce skewness.
- Apply oversampling (SMOTE) or undersampling for imbalanced data.
Use hierarchical classification for better rare-category predictions.
3) When is a nonparametric test used by Data Scientists? Explain its advantages.
Answer:
Nonparametric tests are used when:
- Data does not follow a normal distribution.
- Sample sizes are small.
- Outliers or skewed distributions exist.
Examples:
- Mann-Whitney U Test (comparison of two groups without assuming normality).
- Kruskal-Wallis Test (nonparametric ANOVA for multiple groups).
Advantages:
- Suitable for skewed distributions.
- More robust against violations of assumptions.
- Works with ordinal data or ranked data.
4) What is the role of time series algorithms in Data Science? Explain using a few examples.
Time series algorithms analyze data over time to capture trends, seasonality, and cyclic behavior.
Examples:
- ARIMA (AutoRegressive Integrated Moving Average): Stock price prediction.
- Exponential Smoothing: Forecasting sales for e-commerce.
- LSTMs (Long Short-Term Memory Networks): Demand forecasting in supply chain.
Use Cases:
- Predicting electricity demand in smart grids.
- Sales forecasting for seasonal products.
- Website traffic prediction for better server allocation.
5) Given a random Bernoulli trial generator, how do you return a value sampled from a normal distribution?
Use the Central Limit Theorem (CLT):
- Generate multiple Bernoulli trials (0 or 1 outcomes).
- Sum a large number of these trials.
- Apply mean and variance transformations to approximate a normal distribution.
- Alternatively, use Box-Muller Transform or Inverse Transform Sampling.
Case Study
Problem Statement:
eBay wants to enhance its product recommendation system to provide more relevant and personalized suggestions to users. As a data scientist, your task is to analyze customer behavior, identify patterns, and propose an improved recommendation strategy.
Dataset Overview:
You have access to a dataset containing user interactions and product details. The dataset includes the following attributes:
- User_ID – Unique identifier for each user
- Product_ID – Unique identifier for each product
- Category – Product category (e.g., Electronics, Fashion, Home & Garden)
- Price – Price of the product
- Click_Timestamp – Time when the user clicked on the product
- Purchase_Flag – Whether the user purchased the product (Yes/No)
- User_Search_Keywords – Keywords used by the user to search for products
- Previous_Purchases – List of past purchases made by the user
Key Questions to Answer:
1. How can we identify user preferences for better recommendations?
- What factors influence user engagement with products?
- How do past purchases impact future buying behavior?
- Are there specific patterns in user search queries that can improve recommendations?
2. How can we personalize recommendations for different types of users?
- Should recommendations be based on past purchases, browsing history, or search keywords?
- How can we differentiate recommendations for new users versus returning users?
- Can we cluster users into different segments based on shopping behavior?
3. How can we improve conversion rates through better recommendations?
- What product attributes (price, category, brand) drive the highest sales?
- How can we reduce the number of irrelevant recommendations?
- Can we use real-time data (e.g., current browsing session) to improve recommendation accuracy?
4. What external factors influence user buying decisions?
- Do seasonal trends affect user preferences?
- How do discounts and promotions impact product recommendations?
- Is there a correlation between product reviews/ratings and conversion rates?
For any information related to courses or e-books, please send an email to [email protected]